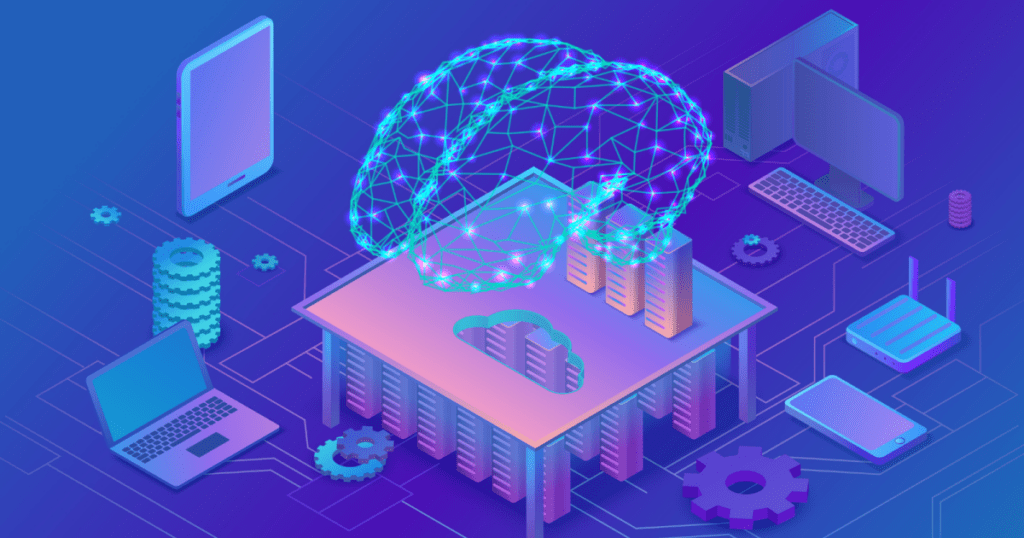
Humpty Dumpty, in “Through the Looking Glass,” says, “When you use a word it means exactly what I want it to mean — neither more nor less.” Alice responds, “The question here is whether words can mean so many things.”
Research has been ongoing on the meaning of words. The human mind must process a vast network of flexible and detailed information in order to understand its meaning.
Word meaning is now being discussed in relation to a new topic. MIT Researchers are looking at how closely artificial intelligence may mirror human brain processes and word understanding. This question has been answered by researchers from UCLA, MIT, and the National Institutes of Health.
The study was published in the journal Nature Human Behaviour. It shows that artificial intelligence systems can pick up complex word meanings. MIT Researchers also discovered a simple way to access this complex information. The AI system that they examined represented word meanings in a way that was very similar to human judgment.
Over the past decade, the AI system developed by the authors has been extensively used to analyze word meanings. It “reads” huge amounts of information on the internet to find word meanings, and it can do so by reading billions of words.
The system recognizes when words are frequently used together, such as “table” or “chair”, and it learns that they have similar meanings. It also learns that pairs of words are not often used together, such as “table” or “planet,” but they can have different meanings.
This seems like a good starting point. But think about how humans would be able to comprehend the world if they could only count the number of words that occur next to each other without the ability to interact with others.
Idan Blank, an assistant professor at UCLA of psychology and Linguistics and co-lead author of the study, stated that the MIT researchers wanted to find out what the system knew about the words it learned and what “common sense” they had.
Blank stated that the research was not complete before Blank explained that the system had one limitation. “Every two words only have one numerical value to represent how similar they are.”
Human knowledge, however, is far more complex and detailed.
Blank stated, “Consider our knowledge about dolphins and alligators.” They are very similar when we compare them on a scale of sizes, from small to large. They are quite different in terms of intelligence. They are quite different in terms of the danger they present to us. The context of a word’s meaning is crucial.
“We wanted to find out if this system is able to recognize these subtle differences, and if its concept of similarity can be adapted in the same way that it is for people.”
The authors devised a method called “semantic project” to find out. One can draw a line from the model’s representations for the words “big”, and then see where different animal representations fall on that line.
The scientists used this method to study 52-word groups to determine if the system could sort meanings. This included judging animals by their size, how dangerous they are, and classifying the U.S. States by their overall wealth or weather.
The other word groupings comprised words for things like initial names, legendary animals, occupations and sports, apparel, and so on. Multiple settings or dimensions, such as size, danger, intellect, and age, were provided to each group.
MIT Researchers found that their method was very similar to intuition across all contexts and objects. (For this comparison, researchers asked 25 individuals to assess each 52-word group in a similar way.
The system was able to see that “Betty” (and “George”) are very similar in that they are both relatively “old,” but were of different genders. The system also discovered that while “weightlifting”, and “fencing” are both indoor activities, they have different intelligence requirements.
Blank stated that it is a simple and intuitive method. Blank said, “The line between big’ and small’ is like an intuitive mental scale. We put animals on this scale.”
Blank stated that he didn’t anticipate the technique would work but was thrilled when it did.
He said, “It turns out this machine-learning system is smarter than we thought. One may learn a lot by observing the words that frequently come together in a language. It includes extremely complicated types of knowledge that are structured into a very logical framework.