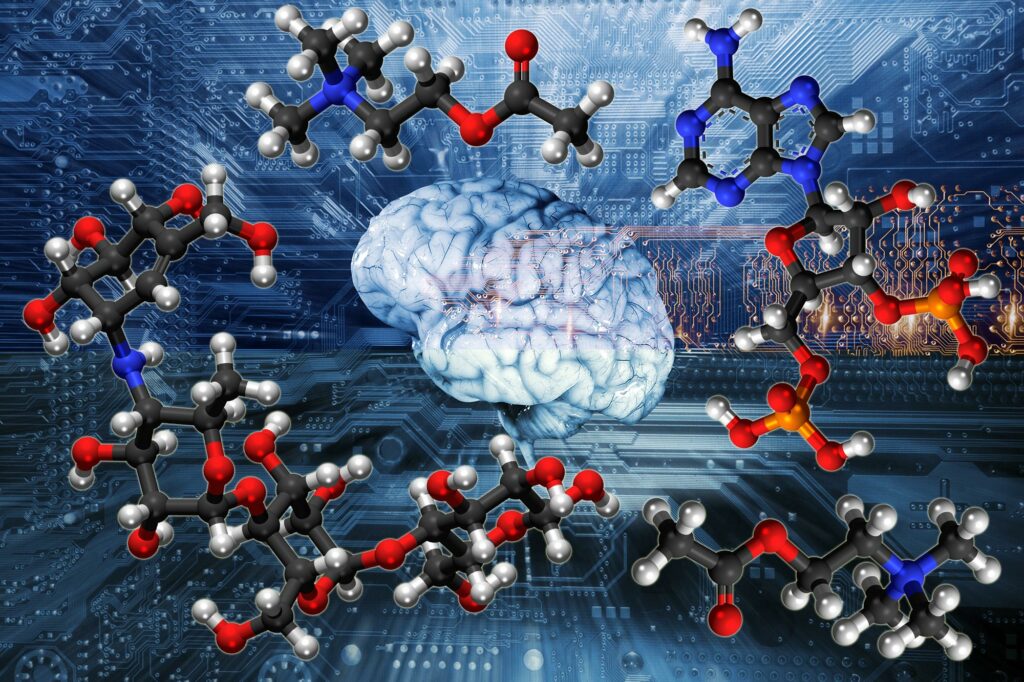
The universe is home to an infinite number of molecules. What percentage of these molecules might have drug-like potential that could be used for life-saving medications? Millions? Billions? Trillions? Novemdecillion is 10 times the power of 60. This number is much greater than what current drug-design models can calculate. It prolongs drug research for fast-spreading conditions like Covid-19.
International Conference on Machine Learning (ICML) will host a paper by MIT researchers. It describes EquiBind’s geometric deep learning model that was developed at MIT and how it successfully bound drug-like compounds and proteins. This is 1,200x faster then QuickVina2-W. EquiBind improves upon its predecessor EquiDock. This technique binds to two proteins using a method that was developed by Octavian Eugen Gagen, a postdoctoral fellow at MIT Computer Science Artificial Intelligence Laboratory and Abdul Latif Jamel Clinic of Machine Learning in Health (Jameel Clinic), and co-author of EquiBind.
Before drug research can begin, drug developers must identify drug-like molecules capable bind or “docking” onto protein targets. This is known as drug discovery. Once the binding agent has been successfully docked with the protein, it can be called the “ligand” which can prevent the protein from functioning. If the binding agent is an essential part of a bacteria, it may stop a protein’s functioning. This will protect our bodies.
The process of drug discovery can be expensive, both in terms of financial costs and computational costs. It takes more than 10 years to develop and test drugs before they can be approved for marketing by the Food and Drug Administration. 90% of drugs tested on humans fail to have any side effects. Drug companies can make up the difference by increasing the price of those drugs that have proved successful.
The current computational process used to find promising drug candidate molecules is as follows: The state-of-the-art computational model relies heavily upon candidate selection and methods such as scoring and ranking in order to obtain the best “fit”.
Hannes Stark, a first-year student in the Department of Electrical Engineering and Computer Science at MIT, is the lead writer of this paper. He compares typical ligand and protein binding methods to “trying to put a key in an open lock with a lot”
EquiBind doesn’t require multiple attempts to locate a ligand-friendly position in a protein like other models. It already uses geometric thinking to aid it in learning the underlying mechanics and making accurate predictions when confronted with new data.
These findings caught the attention of industry professionals quickly, including Pat Walters (chief data officer at Relay Therapeutics). Walters suggested Walters and his team build a model and test existing drugs and proteins for treating lung cancer, leukemia, and other illnesses.
EquiBind was capable of successfully binding these ligands despite the failures of traditional docking techniques.
EquiBind is a unique solution that solves docking problems. It incorporates both pose predictions as well as binding location identification,” Walters stated. This approach makes use of information from thousands upon thousands of publicly available crystal structures. It has the potential to have a significant impact on the field.
Stark said that EquiBind was capable of placing the money in the right spot. This was something we were truly amazed by.
EquiBind was able to get a lot of feedback from professionals in their industry which helped them consider practical uses of the computational model. Stark is looking forward to hearing different perspectives from the ICML in August.
He states that he is interested in getting feedback on the model’s development. “I would love the opportunity to meet with them, to share my ideas about the next steps, and encourage them in using the model for their papers. We have already received many inquiries from researchers asking whether the model can help them.
This work was partially supported partly by the synthesis Consortium & Pharmaceutical Discovery; DARPA accelerated Molecular Discovery and Jamel Clinic; DTRA Discovery of Medical Countermeasures Against New & Emerging Threats program.
This is a tribute to Octavian Eugen Ganea. He was a brilliant scholar and mentor to many of his students.